Nonprofits are Finding New Ways to Get the Data They Need
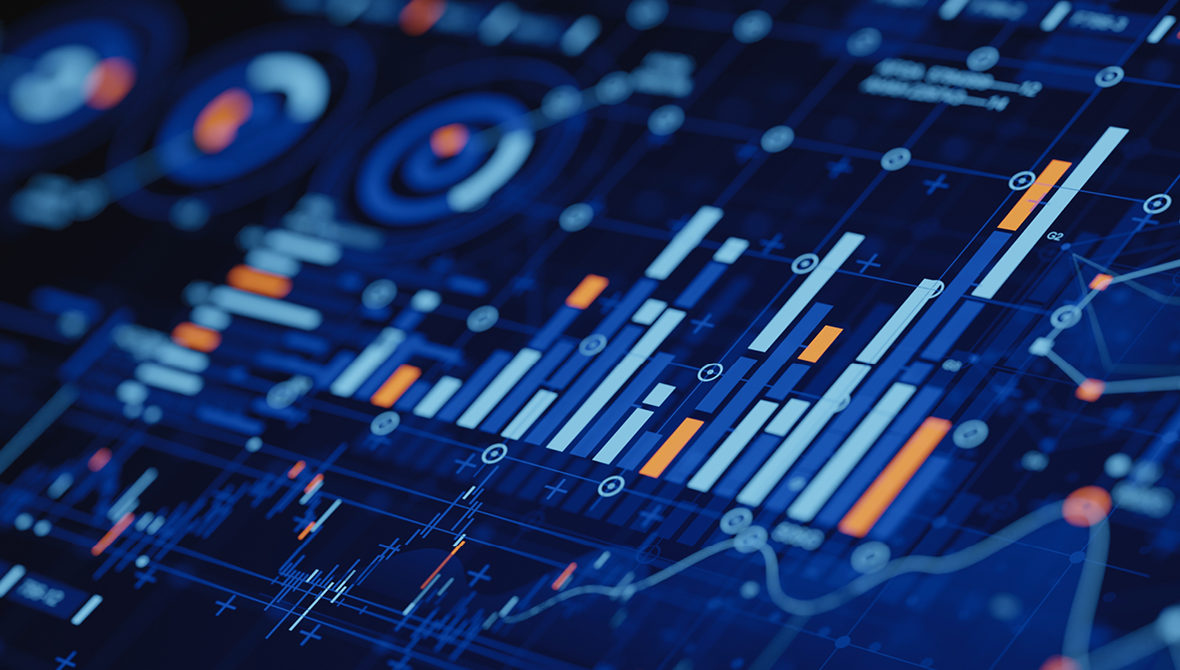
Want the latest trends, research, and more delivered right to your inbox? Subscribe to the Johnson Center email newsletter.
The nonprofit sector has long differed from the for-profit sector in its relationship to data. Benetech’s founder and CEO, Jim Fruchterman, highlighted these differences in the Stanford Social Innovation Review: whereas for-profit entities can harness transactional data to demonstrate financial viability, nonprofits have the unique challenge of collecting data from a variety of sources — communities, staff, donors, policymakers — to demonstrate impact (2016).
Simultaneously, limited budgets and data know-how have held nonprofits back for years (Paz, 2020). To overcome these challenges, many organizations have traditionally relied on existing programmatic data or publicly-available datasets for information about the communities they serve.
“[W]ith the availability of new technology and new partnerships, nonprofits are taking advantage of the rise of data philanthropy, predictive analytics, and machine learning to demonstrate impact.”
However, with the availability of new technology and new partnerships, nonprofits are taking advantage of the rise of data philanthropy, predictive analytics, and machine learning to demonstrate impact.
For decades, nonprofits have especially relied on data and reports made available by government entities. The U.S. Census Bureau’s American Community Survey (ACS) is a prime example. The ACS provides detailed population and housing information (e.g., educational attainment, housing burden, unemployment estimates, etc.) at a variety of geographic levels on an annual basis.
Today, the number of publicly-available datasets — from government and non-government sources — is growing. Part of this is due to an increase in “open data” efforts, such as OpenCorporates (launched in 2010), which shares data on corporations worldwide, and Open990, which takes advantage of the 2019 Taxpayer First Act requiring nonprofits to file their IRS Forms 990 electronically, making hundreds of millions of data points on the nonprofit sector available to the public.
The number of data dashboards is also on the rise (Bauer & Borashko, 2020). Organizations like Data Driven Detroit, Western Pennsylvania Regional Data Center, and the Johnson Center are developing public dashboard platforms. These increasingly powerful tools to extract and display information about specific populations are making it simpler for everyday users to find and display the information they need without having to collect it themselves.
The for-profit sector sits on massive quantities of data about consumer habits, personal mobility, social network behaviors, etc. Some companies are now seeing the potential to use those databanks for more than commercial pursuits.
Data philanthropy allows nonprofits to use private data, otherwise unavailable to them, to better serve their communities. The Mastercard Center for Inclusive Growth is a large promoter of data philanthropy. In one partnership with the Urban Institute, the Mastercard Center for Inclusive Growth provided anonymized and aggregated credit card transaction data, delimited by time and geography (Gerlin, 2018). Researchers at the Urban Institute used that data to investigate charitable giving and equitable development in U.S. cities (McKeever et al., 2019).
Data philanthropy is also playing a large role in the global pandemic response. Many mobile devices track users’ geographic location. As a result, it is possible for private companies like Google to collect mobility data. In 2020, Google started producing COVID-19 Community Mobility Reports. They show how visits to places, such as parks or grocery stores, are changing in frequency, volume, and time of day for different geographic regions.
UNICEF is using these reports — in conjunction with other private datasets from Telefonica, IBM, Amadeus, and Red Hat — to invest in a collaborative platform called MagicBox. MagicBox allows UNICEF to study the socio-economic impacts of physical distancing policies in real time (Garcia-Herranz et al., 2020).
Kirstie Tiernan and Gurjeet Singh (2019) define predictive analytics as, “a set of techniques and technologies that extract information from data to identify patterns and predict future outcomes” (para. 2).
One area where predictive analytics is gaining traction is fundraising and development. Typically, nonprofits must decide who of their prior and potential donors to contact for fundraising. This can be a time-consuming process. Nonprofits must look at donor records and decide which factors appear the most relevant. They may spend time conducting their own statistical analysis on large volumes of data to find this information or contract out the service to a third party.
“One area where predictive analytics is gaining traction is fundraising and development.”
Predictive analytics can streamline this process. Each donor can be assigned a donation probability (using a predictive model), based on historical giving patterns and other donor characteristics. If a donor has a high donation probability, they can be targeted in fundraising campaigns. Using predictive analytics cuts down on the overhead nonprofits spend contacting donors who are unlikely to contribute investments. It also increases the efficiency of fundraising campaigns (Tiernan & Singh, 2019).
In fact, a 2021 Salesforce Nonprofit Trends Report indicated nonprofits who had higher digital maturity, defined as “[the] ability to leverage data to inform decision-making, reach new audiences, personalize communications, and forecast fundraising income” (p. 1), were more likely to meet their fundraising revenue goals.
Some nonprofits are taking predictive analytics a step further with artificial intelligence (AI) and machine learning. Authors France Hoang and Dave Levy (2020) write,
AI makes predictive analytics for nonprofits faster, better, and more affordable. AI can sort through more massive volumes of data than any human could and, through machine learning, develop predictive models faster and with far more complexity and power than what a person could do on their own. (para. 8)
PATH, a Los Angeles-based nonprofit addressing homelessness, provides an example (Levy, 2021). PATH used machine learning to develop LeaseUp, a platform that identifies available housing units in a given area so that case managers can recommend the best housing option in real-time to their clients. Using this technology, PATH has been able to reduce the time it takes to find housing for their clients from 90 days to 45 days.
Although AI and machine learning can be powerful tools, nonprofits should also consider the risks involved with this technology. Anyone working with data must be cognizant of the possible impacts of implicit (and explicit) biases in the information they extract or programs they build (Lee et al., 2019).
It’s also possible for machine-learning programs to make mistakes if human oversight is taken out of the equation (Szalavitz, 2021). Great care should be taken when pursuing AI and machine learning. The rewards can be powerful for an organization, but they do not come without risks.
Bauer, K., & Borashko, R. (2020, January 15). Equity mapping tools combine the power of data and narrative change. 11 trends in philanthropy for 2020. Dorothy A. Johnson Center for Philanthropy. https://johnsoncenter.org/blog/equity-mapping-tools-combine-the-power-of-data-and-narrative-change/
Data Drive Detroit. (2018). Metro Detroit’s community data hub. https://datadrivendetroit.org/
Dorothy A. Johnson Center for Philanthropy. (2021). Your community. Powerful insight. https://data.johnsoncenter.org/community-insight
Fruchterman, J. (2016). Using data for action and for impact. Stanford Social Innovation Review, 14(3), pp. 30–35. https://doi.org/10.48558/24MM-J709
Garcia-Herranz, M., Martini, A., Sekara, V., & Kim, D.-H. (2020, October 10). COVID-19 MagicBox report. UNICEF. https://www.unicef.org/innovation/media/14331/file
Gerlin, A. (2018, August 2). Data philanthropy offers new avenues for solving old problems. Mastercard Center for Inclusive Growth. https://www.mastercardcenter.org/insights/data-philanthropy-offers-new-avenues-solving-old-problems-report-finds
Google. (2021). COVID-19 community mobility reports. https://www.google.com/covid19/mobility/
Hoang, F. (2020, February 24). How artificial intelligence improves predictive analytics for nonprofit fundraising. NonProfit PRO. https://www.nonprofitpro.com/article/how-artificial-intelligence-improves-predictive-analytics-for-nonprofit-fundraising/
IRS. (2019, December 13). IRS: Recent legislation requires tax exempt organizations to e-file forms. https://www.irs.gov/newsroom/irs-recent-legislation-requires-tax-exempt-organizations-to-e-file-forms
Lee, N. T., Resnick, P., & Barton, G. (2019, May 22). Algorithmic bias detection and mitigation: Best practices and policies to reduce consumer harms. Brookings. https://www.brookings.edu/research/algorithmic-bias-detection-and-mitigation-best-practices-and-policies-to-reduce-consumer-harms/
Levy, D. (2021, October 6). How nonprofits are using machine learning to support those in need. NonProfit PRO. https://www.nonprofitpro.com/article/how-nonprofits-are-using-machine-learning-to-support-those-in-need/
McKeever, B., Greene, S., MacDonald, G., Tatian, P. A., & Jones, D. (2018, July 24). Data philanthropy: Unlocking the power of private data for public good. Urban Institute. https://www.urban.org/research/publication/data-philanthropy-unlocking-power-private-data-public-good
Open990. (2021). 1.9 million nonprofits. Zero paywalls. https://www.open990.org/
OpenCorporates. (2021). The largest open database of companies in the world. https://opencorporates.com
Paz, A. (2020, January 15). Data science for social impact. 11 trends in philanthropy for 2020. Dorothy A. Johnson Center for Philanthropy. https://johnsoncenter.org/blog/data-science-for-social-impact/
Salesforce. (2021). Nonprofit Trends Report (3rd ed.). https://www.salesforce.org/wp-content/uploads/2021/01/ngo-trends-report-third-edition-122120-v2.pdf
Szalavitz, M. (2021, August 11). The pain was unbearable. So why did doctors turn her away? WIRED. https://www.wired.com/story/opioid-drug-addiction-algorithm-chronic-pain/
Tiernan, K., & Singh, G. (2019, January 10). Predictive analytics. The NonProfit Times. https://www.thenonprofittimes.com/column_database/predictive-analytics/
Western Pennsylvania Regional Data Center. (2017). Tools. https://tools.wprdc.org/
U.S. Census Bureau. (2021). American Community Survey (ACS). https://www.census.gov/programs-surveys/acs